New analysis allows accurate prediction of slaughter age
Research published in the Journal of Animal Science has shown analysis method can accurately use phenotype and gene data to predict the age at which pigs will be ready for slaughter.We are all aware that AI and machine learning are making precision agriculture ever more sophisticated. Now, scientists at the Bernoulli Institute for Mathematics, Computer Science and Artificial Intelligence at the University of Groningen in the Netherlands are using artificial intelligence to predict the age at which pigs will be ready for slaughter (that is, exactly when each pig will reach its target weight).
A recent study by Ahmad Alsahaf, George Azzopardi, Bart Ducro, Egiel Hanenberg, Roel F Veerkamp and Nicolai Petkov examining the accuracy of phenotypic and genetic data in predicting slaughter age was prompted by the fact that correctly forecasting the growth of pigs can help determine things like feed costs and pen space requirements. Growth-rate predictions could also be used in other ways – for example, to identify which growth-rate factors might be more important than others.
However, the scientists note that making growth-rate predictions is not easy to do, due to natural variations in the way individual pigs grow. To tackle the problem, the team used machine learning – specifically a statistical analysis called random forest (RF) regression, to examine data on individual pigs.
They chose this particular analysis, says Alsahaf, as it’s been a popular algorithm in machine learning applications for a long time and is suited to the purpose of the study.
“It belongs to a family of algorithms which are based on decision trees,” he explains. “These algorithms are well suited for prediction problems that have heterogenous input types, as is the case with our data. The other advantage is that the method is conceptually easy and the results are simple to interpret due to the ‘importance scores’ that the method produces.” However, he notes that other equally effective analysis methods could have been used.
Alsahaf and Azzopardi also used the “variable importance score” derived from RF regression to measure the importance of the different types of input data in making the prediction.
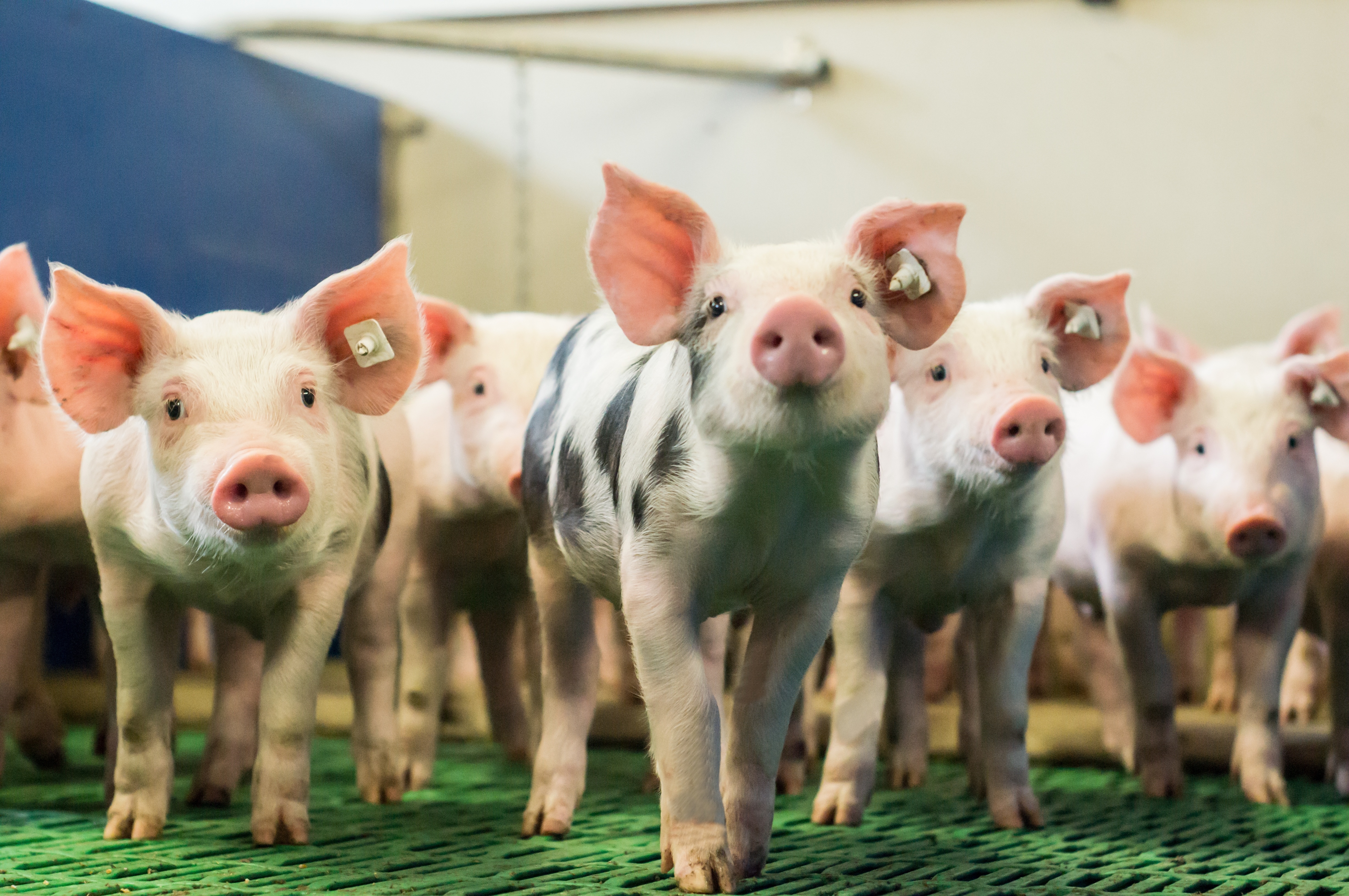
Study details
The study involved individual weight measurements at different growth stages on almost 33,000 individual purebred Large White pigs, data which was provided by the major breeding firm that owned the pigs. The scientists also used the firm’s phenotypic data (such as late weight measurements, sex of the pig, litter information and birthweight relative to litter average), estimated breeding values (EBVs) and pedigree and pedigree-genetic relationships.
The specific prediction of the study (otherwise known as the output) was the age at which an individual pig would reach a slaughter weight of 120kg. Its results – comparing the researchers’ statistical analysis with the actual age at which each animal reached 120kg – showed that, overall, the method’s level of accuracy was quite high.
“In our experiments, this accuracy corresponds to predicting slaughter age within nine days of the true value on average (a mean absolute error of 8.48 days to be precise),” says Alsahaf. “To our understanding, this can already provide a logistical advantage compared to the current standards.”
The study also showed that phenotypic features such as weight measurements (at birth, weaning and at the start of the fattening stage) were the most effective in predicting the target output; these features explained approximately 62 percent of the output variance.
“We chose the features we did mainly for their potential relevance, determined by experts in the field, to the output we wanted to predict,” Alsahaf say. “Our choice was also limited to data that had been routinely collected already for those animals.” Individual feed consumption data, for example, was not available.
Results as expected?
Alsahaf explains that growth prediction at the individual pig level is a new area of study and that he hopes future studies can improve upon his team’s work. “In my opinion, the differences in accuracy at this stage come down to differences in experiment design and available data more so than the particular prediction method used,” he notes. “I think the framework we proposed, using a combination of phenotypic and genetic data, is an effective one for these kinds of prediction problems.”
According to Alsahaf, the inclusion of additional relevant data, which would have to be collected on a large scale, will improve the prediction accuracy – in particular, more weight measurements at different stages of the pig’s life and corresponding feed intake measurements would be expected to enhance the precision of the growth-rate forecasts.
When asked how much production practices such as early care, temperature and air-quality control have an effect on commercial pig growth rates, Alsahaf notes that he personally can’t comment as it’s not something he’s studied. “I think it’s an interesting topic to explore in future research,” he says, “but collecting the data in such a way that ensures its uniformity between different farms and locations would prove to be a challenge in itself.”
Contacts for publication records
Ahmad Alsahaf University of Groningen, Bernoulli Institute for Mathematics, Computer Science and Artificial Intelligence, AK Groningen, The Netherlands [email protected]
This research is part of the TTW-B4F project.
All data was provided by Topigs-Norsvin.